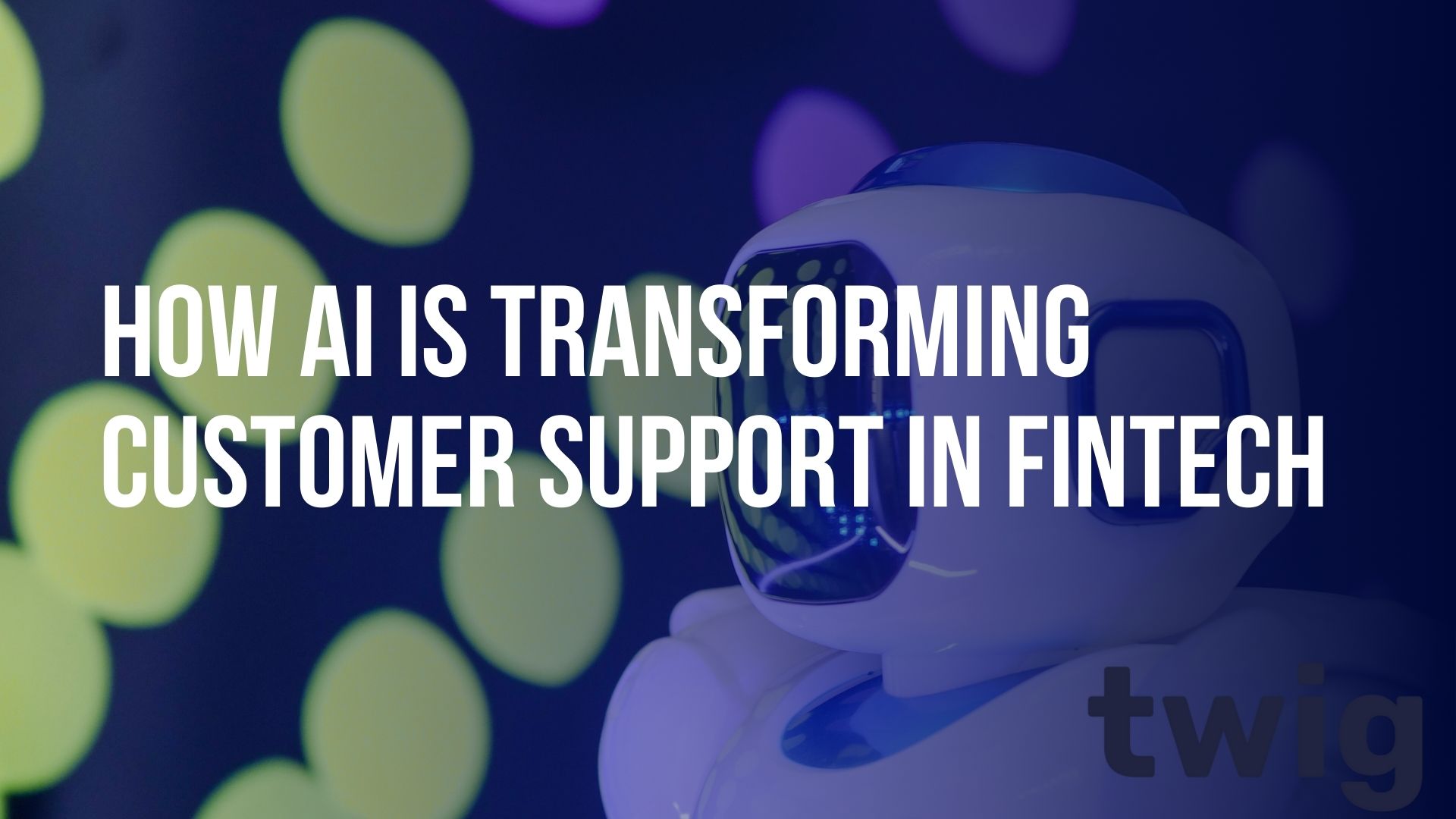
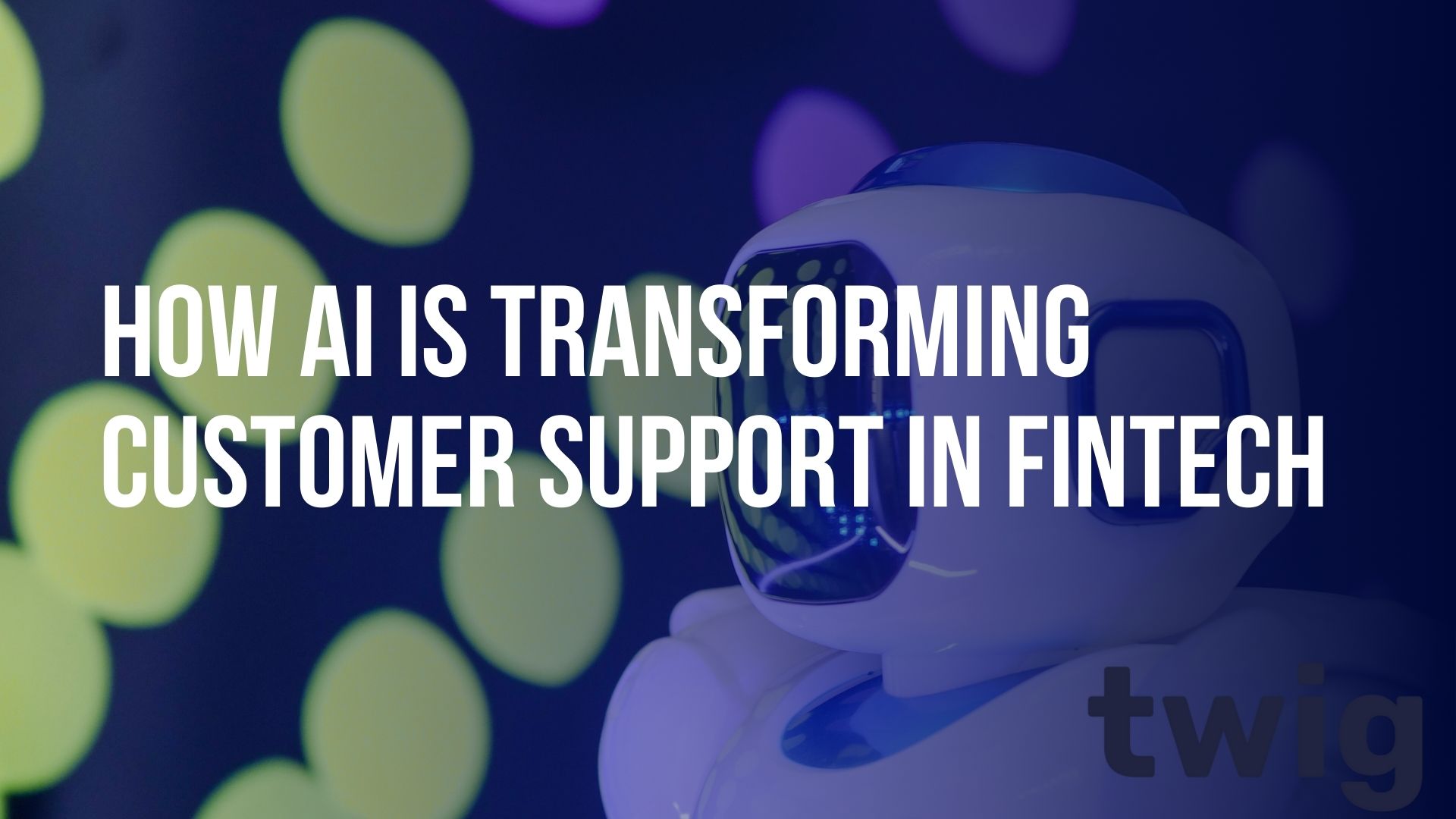
Fintech success is increasingly defined by one thing: how fast and accurately you can predict what happens next—from market fluctuations to customer behavior shifts.
Traditional analytics focuses on hindsight. But in today’s high-stakes financial environment, reaction isn’t enough. Fintech firms need systems that forecast, suggest, and act, ideally before problems or opportunities even appear.
Enter AI-powered predictive analytics—a technology stack that combines behavioral data, deep learning models, and real-time feedback to help teams move from dashboards to decisions in milliseconds.
A 2023 Deloitte report found that predictive analytics increased fintech firms' marketing ROI by up to 25%, while improving customer retention by over 30% in high-churn segments.
AI-driven predictive analytics isn’t just a tool for hedge funds or trading desks. It’s now being embedded in customer service platforms, onboarding flows, credit modeling, and churn prevention systems.
Key applications include:
Unlike legacy analytics systems, AI doesn’t just identify trends—it learns patterns, adapts to changes, and makes recommendations automatically.
The predictive power of AI platforms lies in deep learning—neural network models trained on massive, multi-dimensional data sets.
What sets deep learning apart?
So instead of reacting to a drop in monthly active users, a fintech company can proactively engage the users most at risk of churn—with tailored offers, interventions, or personalized outreach via AI assistants.
That’s the shift from reporting to real-time strategic action.
The best predictive analytics systems today don’t live in spreadsheets—they live inside your customer workflows.
Modern agentic AI assistants can:
These AI agents are evolving from passive tools into co-pilots—surfacing predictive insights in real time, not after the fact.
A VP of Customer Success at a neobank can now get alerts like:
“Users in Segment C with >3 declined payments and no login in 7 days have a 78% churn risk. Recommend sending re-engagement offer.”
This isn’t theory—it’s already in action at fintechs like Wise and Chime, which leverage behavioral models to predict churn and reduce CAC through smart retention flows.
Beyond individual customer behavior, AI is helping fintech firms read the market itself.
Using techniques like:
AI systems can flag emerging risks or opportunities faster than human teams ever could.
A 2024 Gartner study noted that fintechs using predictive analytics in treasury and risk ops achieved 2x faster response time to market-moving events and 15–20% reduction in exposure-related losses.
Predictive analytics is most powerful when deeply integrated—not tacked on.
Here’s where it connects:
When predictive insights are embedded at every layer, AI becomes part of the value proposition, not just a reporting function.
Of course, AI-powered forecasting must be auditable, explainable, and fair.
Today’s best AI platforms include:
This is especially important in credit, lending, and risk decisioning—where a misstep can hurt both customers and reputation.
In a world of infinite fintech apps and platforms, forecasting intelligently is what sets market leaders apart.
Predictive AI empowers CX teams, support agents, and product leaders to:
Fintech leaders investing in predictive analytics aren’t just solving today’s problems—they’re preparing for tomorrow’s.