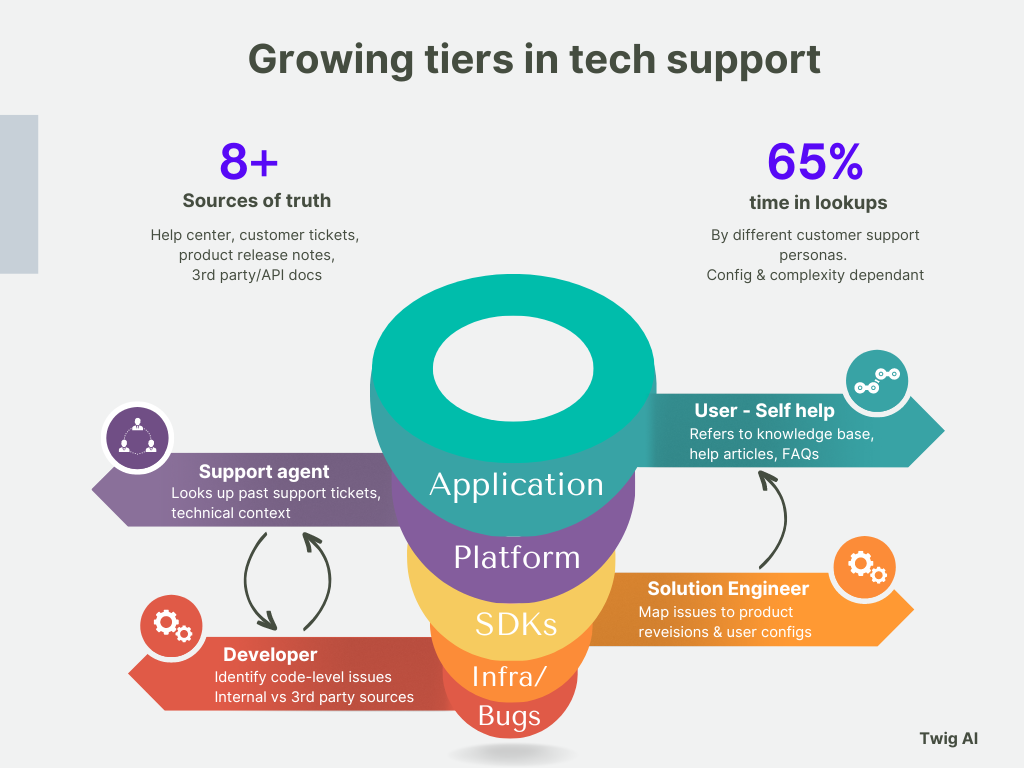
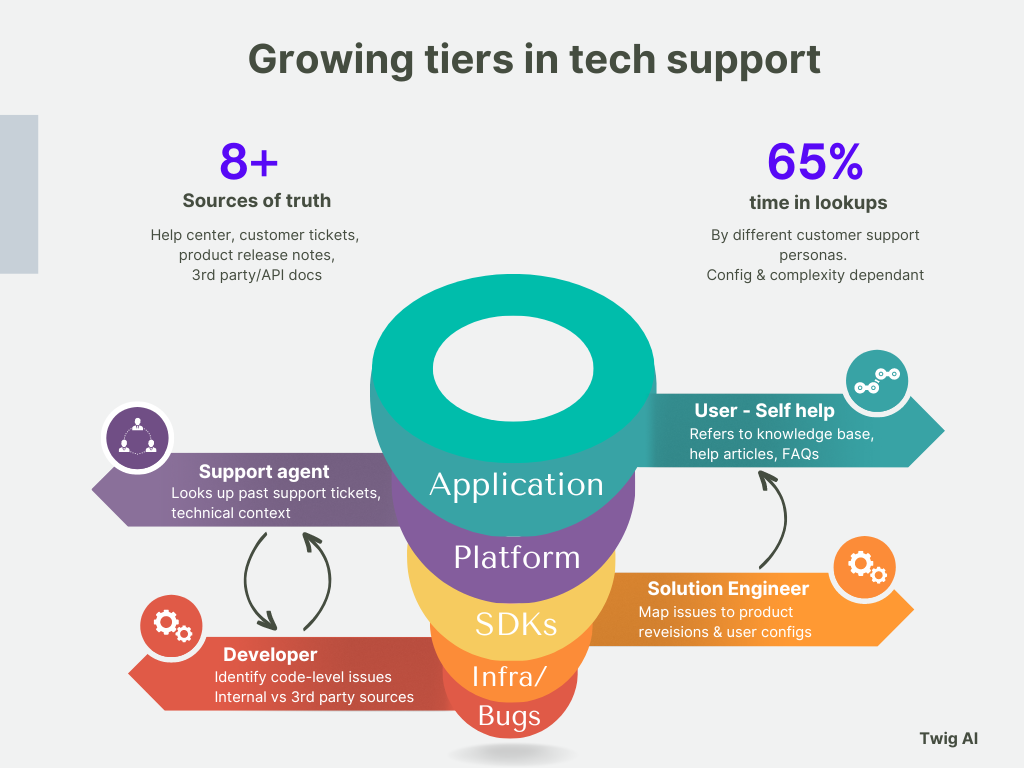
While we're aware how artificial intelligence (AI) technologies is going to change fundamental aspects of supporting customers, it's important know key differences in AI adoption for Technical support vs. general customer support.
This lets us uncovering unique requirements for technical solutions and complex products when delivering efficient and personalized experiences with AI.
It's the long tail of technical issues which are usually context based, unique to each product feature and different customer settings which needs a different approach to bring AI efficiency.
While common workflows of AI-powered customer solutions automate repetitive tasks, provide instant assistance through chatbots, and self-service portals.
AI for tech support has completely new way to automate covering different modes of operation (app + APIs), different user bases (highly skilled developer and professional users), and varied revisions of product SKUs.
This article explores how AI redefines specialized technical support and general customer support, highlighting key benefits like cost savings, faster response times, and agent efficiency.
We'll examine few AI applications of technical support, uncovering their differences, and explore implementation considerations for leveraging AI in these domains.
Technical support for any software or tech devices include understanding full context of customer, analyzing the scenario often with help of logs or application settings, root causing issues following run books, and finally suggesting a change or fixing the bug. This is what usually followed by support agent or tech engineers.
While most support AI's automates basic informational type of queries by referencing knowledge base, help docs. Many tech teams see challenge getting a full-stack of AI solution for their complete ticket triage.
As an example here's how increasingly complex software products require agents to go through. From Application level data and configuration to Platform, SDK documents to analyze, Eco-system and infra issues to known bugs and work arounds.
This naturally impacts customer experience and KPIs like first response time usually over 15 minutes and ticket handling rate which is going upward 24 hours, for many well known tech companies.
Some key capabilities include:
While AI and advanced GPTs have capabilities to bring efficiency it's imperative to think about requirements which are unique to tech support. Talking to support leaders and tech agents in B2B space here're few points we've gathered
An example is given below, with AI responses showing exact citation from where answers were sourced. Also referring to only trusted resources within the org, not from outside information (Google, ChatGPT). And ability to keep the answers current and update to agent-in-the-loop feedback.
In the dynamic world of technology, flexibility and adaptability are key. That's why tech support teams seek AI solutions that offer better control and customization.
Quality of data is the cornerstone of problem-solving in AI. However, enterprises often face challenges due to the limitations of available data. Delivering effective results within these data constraints is what expected from tech support AI
AI-powered customer support solutions excel at handling routine queries and tasks, allowing human agents to focus on more complex issues. Some key capabilities include:
While AI has transformed general customer support, it's important to strike a balance between automation and human interaction. Customers often appreciate the empathy and personalized attention that human agents can provide, especially in complex or emotionally charged situations.
The key difference between customer service and technical support lies in the level of expertise required. Customer service teams act as the face of the company, focusing on building meaningful relationships with customers and ensuring a positive overall experience. They handle general inquiries, complaints, and issues related to products or services.
On the other hand, technical support requires a higher level of specialized knowledge and expertise to troubleshoot and resolve specific technical problems. Technical support agents must have in-depth understanding of the products or systems they support, and their work is more reactive in nature, addressing issues as they arise.
Customer service teams have a broader focus on the overall customer relationship and experience. Their goal is to create a positive impression of the company and foster long-term customer loyalty.
In contrast, technical support teams have a narrower focus on resolving technical issues and ensuring the proper functioning of products or systems. Their primary objective is to provide effective solutions to technical problems, enabling customers to fully utilize the products or services they have purchased.
Customer service often involves proactive efforts to anticipate and address customer needs, such as providing product information, offering personalized recommendations, and addressing potential concerns before they escalate.
Technical support, on the other hand, is typically reactive, responding to specific technical issues reported by customers. Technical support agents rely on their expertise and problem-solving skills to diagnose and resolve these issues efficiently.
In this article, we explored how artificial intelligence (AI) is transforming the realms of technical support and customer support. While AI excels in automating repetitive tasks, providing instant assistance, and enabling data-driven insights, the human touch remains invaluable for complex issues that require empathy and deep domain expertise. A balanced approach that harnesses the strengths of both AI and human support can deliver efficient, personalized experiences tailored to customer needs.
Moving forward, companies should strategically integrate AI solutions to streamline operations, reduce costs, and enhance customer experiences. At the same time, investing in human capital, fostering emotional intelligence, and nurturing specialized technical knowledge will be crucial for success. To gain a sneak peek at practical AI use cases, businesses can explore AI-powered tools that seamlessly blend automation and human expertise, unlocking new possibilities in technical support and customer service.
1. How can AI be implemented in technical support?AI can significantly enhance help desk management through various applications such as providing 24/7 support via chatbots, automating ticket sorting, offering self-service knowledge bases, conducting sentiment analysis, routing to the most suitable agent, providing smart suggestions and recommendations, translating languages, and using predictive analytics.
2. What are some ways AI is utilized in customer service?AI is commonly employed in customer service through tools like sentiment analysis chatbots. These chatbots engage in conversations and analyze the tone of the customer using specific phrases. This helps businesses understand customer emotions towards their products and services.
3. What does a customer support system powered by AI entail?An AI-powered customer support system involves chatbots that handle customer inquiries and simulate human-like conversations using natural language processing (NLP). These systems are built on artificial intelligence, machine learning, and natural language understanding (NLU) to replicate human interactions.
4. When did AI first appear in customer service?AI made its initial appearance in customer service in the 1970s with the use of Interactive Voice Response (IVR) systems by bank tellers to check customer account balances. IVR technology has evolved to be much more intelligent and efficient, continuing to serve in various customer service capacities today.
[1] - https://www.zendesk.com/blog/ai-customer-service/
[2] - https://www.salesforce.com/blog/customer-service-ai/
[3] - https://levity.ai/blog/ai-for-customer-support
[4] - https://www.mindmesh.com/glossary/what-is-ai-customer-service
[5] - https://blog.hubspot.com/service/customer-service-vs-technical-support
[6] - https://selectvoicecom.com.au/blog/ai-vs-human-customer-support/
[7] - https://hiverhq.com/blog/ai-vs-human-in-customer-service